Machine Learning, Spring 2024
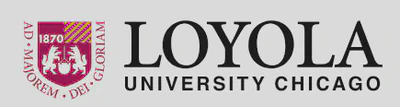
Details
Course: COMP 379-001 / COMP 479-001 Machine Learning
Level: Undergraduate and Graduate
Instructor: Daniel Moreira (dmoreira1@luc.edu)
Lectures: THR, 4:15 to 6:45 PM, 123 Institute of Environmental Sustainability
Office Hours: FRI, 8 AM to 5 PM, 310 Doyle Center or Zoom,
by appointment
Sakai: https://sakai.luc.edu/x/Od0gze
Final grades were released on Sakai. Thank you, everyone!
Overview
“Machine Learning is the field of study that gives computers the ability to learn without being explicitly programmed.”
– Arthur Samuel, 1959
Even though Samuel’s definition is now more than six decades old, it still holds true. With the recent advances in computer processing power, memory, and storage, machine learning has stressed its learn-by-example data-driven aspect, and is available — commonly as a black box — to everyone.
Annotated high-quality datasets can be easily harnessed to train a multitude of models to solve very specific problems under the different paradigms of supervised, unsupervised, hybrid (e.g., semi-supervised and self-supervised), and reinforcement learning. This course will cover these paradigms, trying to establish a balance between theory and practice. While students will be exposed to the theories that fight the black-box and irresponsible usage of machine learning, hands-on activities leveraging real-world data will prepare them for industrial, academic, and societal needs.
Let’s learn how the machines learn!
Requirements to attend this course are basic programming skills (especially Python), data structures, math fundamentals (such as linear algebra and calculus), and probability and statistics. This course and its materials are available in Sakai.
Schedule (materials in Sakai)
- 01/18 - Syllabus and Intro
- 01/25 - Data-driven Aspects
- 02/01 - Data-driven Aspects (cont.)
- 02/08 - Principal Component Analysis
- 02/15 - Linear Regression
- 02/22 - Linear Regression (cont.)
- 02/29 - Logistic Regression
- 03/07 - Spring Break, no classes
- 03/14 - Classification Metrics
- 03/21 - Classification Methods (K-Nearest Neighbors, Decision Trees)
- 03/28 - Easter Break, no classes
- 04/04 - Classification Methods (cont., Support Vector Machines)
- 04/11 - Neural Nets
- 04/18 - Convolutional Neural Nets
- 04/25 - Graduate Students’ Lectures and Wrap-up
- 05/02 - Project Presentation
Important Dates
02/15 - Definition of project groups.02/29 - Definition of project topics.03/07 - Spring Break.03/21 - Midterm Exam.03/21 - Definition of project plan.03/28 - Easter Break.04/11 - Report of project status.04/25 - Graduate students’ lectures.05/02 - Project presentation.
Notebooks (for hands-on activities)
- Notebook 01, Data-driven Aspects.
- Notebook 02, Principal Component Analysis.
- Notebook 03, Linear Regression.
- Notebook 04, Logistic Regression.
- Notebook 05, Classification Methods.
- Notebook 06, Neural Networks.
Grading
Concept | Interval (%) | Concept | Interval (%) | Concept | Interval (%) | Concept | Interval (%) |
---|---|---|---|---|---|---|---|
A | [96, 100) | B+ | [88, 92) | C+ | [76, 80) | D+ | [64, 68) |
A- | [92, 96) | B | [84, 88) | C | [72, 76) | D | [60, 64) |
B- | [80, 84) | C- | [68, 72) | F | (0, 60) |
Distribution
Undergraduate | Graduate | |
---|---|---|
Assignments (3) | 30% | 25% |
Exams |
30% | 25% |
Project | 30% | 25% |
Participation | 10% | 10% |
Topic Lecture | N.A. | 15% |
On the News | +1% (extra) | +1% (extra) |
Assignments (materials in Sakai)
- Assignment 1, Data-driven Aspects, Notebook 01.
- Assignment 2, PCA and Linear Regression, Notebooks 02 and 03.
- Assignment 3, Logistic Regression and Classification Methods, Notebooks 04 and 05.
Late Policy
Deduction of 10% of the maximum possible grade for each day of delay.
Exams
- Midterm Exam, 03/21, good answers.
Final ExamFinal Project Presentation, 05/02.
Project
- Written report (and presentation), work alone or in groups.
Chosen Topics
Group | Topic | Group | Topic |
---|---|---|---|
Team 01 | Breast Cancer Detector | Team 11 | March Madness Standings Predictor |
Team 02 | Car Price Estimator | Team 12 | House Price Estimator |
Team 03 | Real Estate Predictor | Team 13 | Music Source Separator |
Team 04 | LUC Ranking Estimator | Team 14 | Soccer Player Transference Value Estimator |
Team 05 | Bitcoin Trading Advisor | Team 15 | Lawyer Recommendation System |
Team 06 | Car Emission Estimator | Team 16 | Music Genre Classifier |
Team 07 | NBA MVP Predictor | Team 17 | Heart Condition Classifier |
Team 08 | Twitter Sentiment Analyzer | Team 18 | Person’s Age Prediction from MR |
Team 09 | Diabetes Condition Classifier | Team 19 | Credit Condition Classifier |
Team 10 | Credit Card Fraud Detector |
Participation
- Class Attendance: every presence counts.
- Today-I-missed Statements: every submission counts.
Today-I-missed Statements
After every attended class, each student will have to submit (through Sakai) a short paragraph answering one of the following:
- What is your biggest question after class? OR
- What was the most interesting point you learned today?
Inspired by Dr. Sandra Avila.
Oopsie Cards
Each student has two “Oopsie” Cards, which will allow them to avoid losing points because of late delivered work. The cards are not valid to dismiss or postpone exams, topic lectures (graduate students), or final project dates. Students may use their cards at their own discretion, as long as they clearly communicate the instructor.
Life happens, be wise.
ML on the News
Posted by the students on Sakai.
- OpenAI Sora.
- Self-driving delivery robots.
- EU AI Law.
- Devin - AI Software Engineer.
- AutoML - Automated ML model development.
- Open-Source Companies Are Sharing Their AI Free. Can They Crack OpenAI’s Dominance?
- BharatGPT Hanooman.
- Remote Brain Surgery Training.
- Figure.ai.
- DALL-E 2: Create realistic images and art from a description in natural language.
- GFP-GAN : Photo Restoration.
- Machine Learning Uncovers New Ways to Kill Bacteria With Non-Antibiotic Drugs.
- To build a better AI helper, start by modeling the irrational behavior of humans.
- Meet Your New Assistant: Meta AI, Built With Llama 3.
Links
- Python Machine Learning book, code repository.
- Hands-on Machine Learning with Scikit-Learn, Keras and TensorFlow book, code repository,
- An Introduction to Statistical Learning with Applications in Python book, online version.
Academic Integrity
Students are expected to adhere to the LUC statements on academic integrity available at https://bit.ly/3TmiQkQ. These policies fully apply to this course. The penalty for task-wise academic misconduct is losing all the task’s points. Multiple events of misconduct will incur in failing the entire course (with an F grade). All cases of academic misconduct will be reported to the proper department offices. Lastly, students are not allowed to use AI assisted technology (such as ChatGPT) along the entirety of the course, unless explicitly authorized by the instructor.
Accommodations
Students who have disabilities and wish to request academic accommodations are advised to contact the Services for Students With Disabilities (SSWD) office at 773-508-3700 or SSWD@luc.edu as soon as possible. The SSWD office will provide accommodation letters that, once shared with the instructor, will be fully accommodated as per the terms of their content with no further questions.
Acknowledgements
I would like to sincerely thank Professors Sandra Avila and Dmitriy Dligach for kindly sharing their Machine Learning course materials with me. I relied upon their content and experience to constitute this course.